Demystifying AI ROI: A Simple Framework for Business Leaders
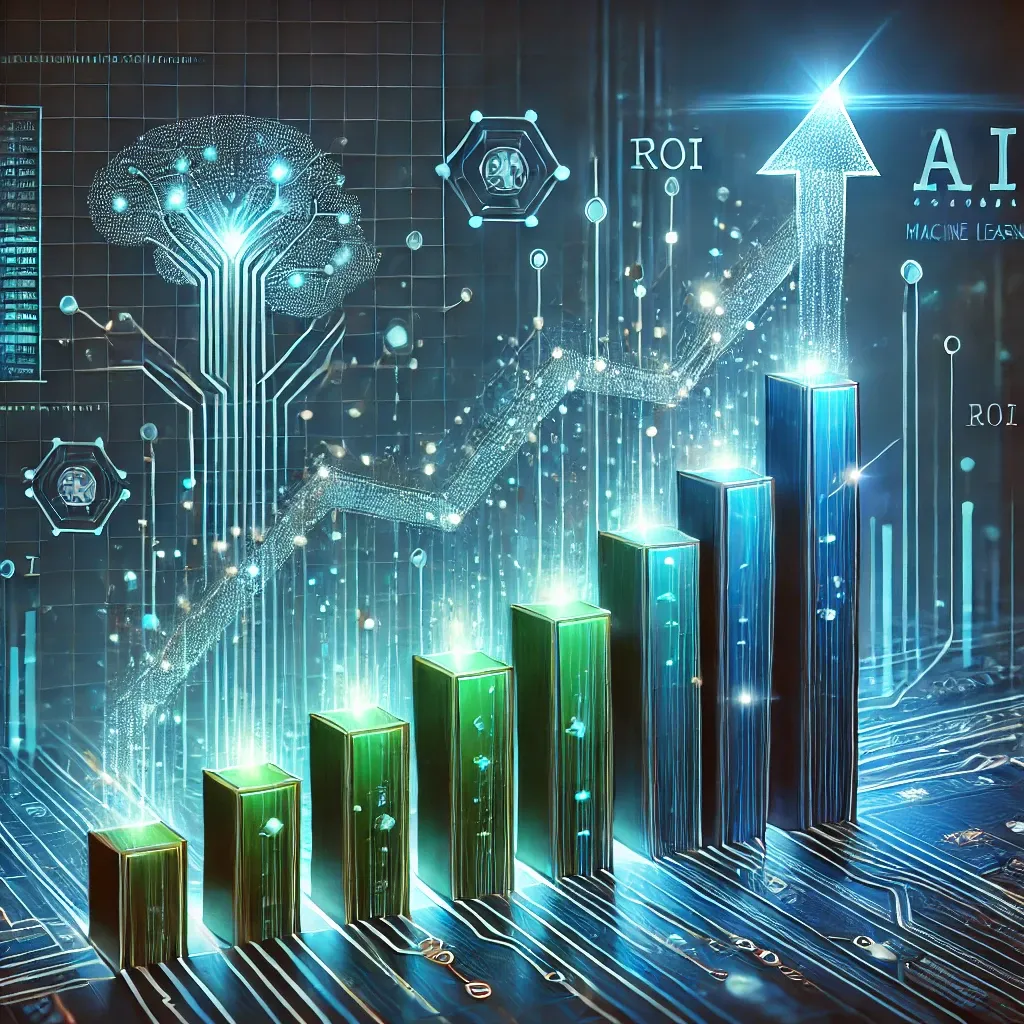
Artificial Intelligence is everywhere—buzzwords flying, companies scrambling, and business leaders wondering if they need to “do AI” before their competitors outpace them. But here’s the real question: Is AI actually useful for your business?
This is where things get messy. Many leaders get caught up in the wrong discussions—debating the cost-performance ratio of foundation models, getting lost in technical jargon, or assuming AI can magically solve problems without a clear use case. The truth is, AI is not a silver bullet. But it is a powerful tool—if you know how to assess its value properly. You'd be surprised how often I hear about teams implementing an ROI experiment before fully vetting the use case. It happens, trust me.
Instead of drowning in analysis paralysis, here’s a simple step-by-step framework to determine whether an AI use case is worth pursuing.
Step 1: Define the Task, Not the Technology
Don’t start by asking, “How can we use AI?” That’s backwards. Instead, ask: “What task are we trying to improve, automate, or enhance?”
- Is it repetitive and time-consuming? (e.g., research and synthesis, customer service responses)
- Does it involve pattern recognition? (e.g., fraud or anomaly detection, quality control in manufacturing)
- Could AI augment rather than replace human effort? (e.g., summarizing reports, generating content drafts)
For example, AI-powered research and synthesis allow professionals to review multiple sources simultaneously, aligning them to core principles and identifying patterns without the bias or time constraints of manual research.
That said, human intuition still plays a key role in many decision-making processes. Consider something as simple (or maybe as complex) as distinguishing between different meanings. An AI might process words or images similarly, but an individual human’s background, experiences, and implicit understanding of context shape how they interpret and prioritize that information. AI can speed up synthesis, but it doesn't replace the unique insights that come from lived experience. Yes, diversity, equity, and inclusion (DEI) are still critically important.
If the task is well-defined and AI could meaningfully contribute, move to Step 2.
Step 2: Identify the Bottleneck and Consider Task Complexity
AI works best when it removes friction. So, what’s slowing things down?
- Time Consumption – Are critical tasks taking too long? Are expert personnel costly per hour? (e.g., manual expert research that takes weeks or months)
- Volume – Too much data, not enough time? (e.g., scanning thousands of reports)
- Accuracy – Is human error causing issues? (e.g., missing insights in extensive datasets)
However, not all tasks are equally suited for AI automation. Some tasks rely purely on pattern recognition, while others require domain expertise and human intuition to interpret subtle connections that may not be explicitly present in the data. This is where individual background and context come into play.
A sales executive with deep industry experience might recognize patterns in customer behavior that an AI model would miss—because those patterns aren’t always explicit in the data. AI can help surface insights, but humans still bring experience-driven intuition to decision-making.
For more advanced AI adoption, organizations should consider deploying multiple AI agents within a workflow to handle different aspects of a task. Alternatively, where AI cannot fully replace human expertise, a co-pilot strategy should be intentionally designed—AI assists decision-makers in achieving better outcomes rather than merely automating for automation’s sake.
If AI can directly address the bottleneck while accounting for complexity, go to Step 3.
Step 3: Determine the Potential ROI
Now, let’s talk numbers—but in a way that makes sense.
A use case is worth pursuing if AI can deliver at least one of these three benefits:
- Cost Savings – Will it reduce labor or operational costs? You need to define appropriate metrics for this.
- Revenue Growth – Will it create new opportunities for sales or market expansion?
- Efficiency Gains – Will it significantly improve productivity or decision-making speed? Yes, you need to establish metrics here too. See the pattern?
Not all tasks justify AI investment equally. Leaders should assess repetition and frequency to determine impact.
- High-value, long-duration tasks (e.g., synthesizing customer research) often see greater returns because they directly influence strategic decisions.
- Simple, fast tasks (e.g., customer troubleshooting) can yield value only if they occur at high frequency. For instance, automating a five-minute customer service query wouldn’t provide much value if it only happens a few times a day, but at scale—handling thousands of interactions daily—it can significantly reduce operational overhead.
If AI can provide measurable ROI while aligning with frequency and complexity factors, proceed to Step 4.
Step 4: Consider the Effort vs. Impact Trade-Off
AI isn’t free, and implementation takes time. So, weigh effort against potential impact.
- Data Readiness – Does your company have structured, accessible data? Without clean data, AI implementation will fail.
- Low Effort, High Impact – Implement immediately (e.g., AI-driven research synthesis for faster sales insights)
- High Effort, High Impact – Worth exploring but requires investment (e.g., AI-powered customer analytics)
- High Effort, Low Impact – Avoid (e.g., automating something that barely affects business outcomes)
Many businesses get stuck because they lack the data infrastructure to support AI. If the data is there and AI impact outweighs the effort, move to Step 5.
Step 5: Test Before You Invest
Don’t go all in—pilot first. Can you test AI on a small scale with minimal disruption?
- Involve the actual workload team in the pilot.
- Use AI as a co-pilot, rather than a full replacement—assess how AI augments human workflows.
- Set clear accuracy metrics based on existing human performance benchmarks.
- Measure real results against your initial assumptions.
- Iterate based on feedback before full adoption.
Clear expectations are key. Some roles may shift, and certain routine tasks may become automated—but the goal is to free up human effort for higher-value work that fosters innovation, better decision-making, and more fulfilling roles. If the pilot shows tangible results, you’ve found a strong AI use case.
Final Thoughts: Becoming Data-Ready for AI Adoption
Moving forward, businesses need to become data-ready before implementing AI. Leaders should:
- Encourage documentation of tasks to create structured datasets for AI training.
- Set clear expectations for AI’s role, ensuring it supports more productive and efficient workflows.
- Communicate AI’s strategic purpose—highlighting how AI improves outcomes, enhances efficiency, and reduces mundane tasks, allowing employees to focus on meaningful work.
- Invest in training—clear expectations for human roles translate into more effective AI implementation.
Action Item:
Try this framework on a task in your own business. Does AI enhance it? If so, you might just have your next game-changing AI use case.
And if you found this useful, share it—because the world doesn’t need more AI hype. It needs more AI that actually delivers—creating better businesses and happier employees.